Have you ever found yourself caught between awe-inspiring potential and lingering doubts when it comes to Generative AI?
It is an undeniable fact that generative AI is reshaping the tech landscape, offering immense potential for businesses.
However, it may appear as a maze of mysteries where distinguishing between myths and truths can be a daunting task. And you might have done hundreds of Google searches for the same.
Well, your search ends here. In this blog we will bust some myths surrounding it and help you clear the fog in your head so that you can unlock its true potential to grow your business.
So, without wasting much time, let’s get started.
What is Generative AI?
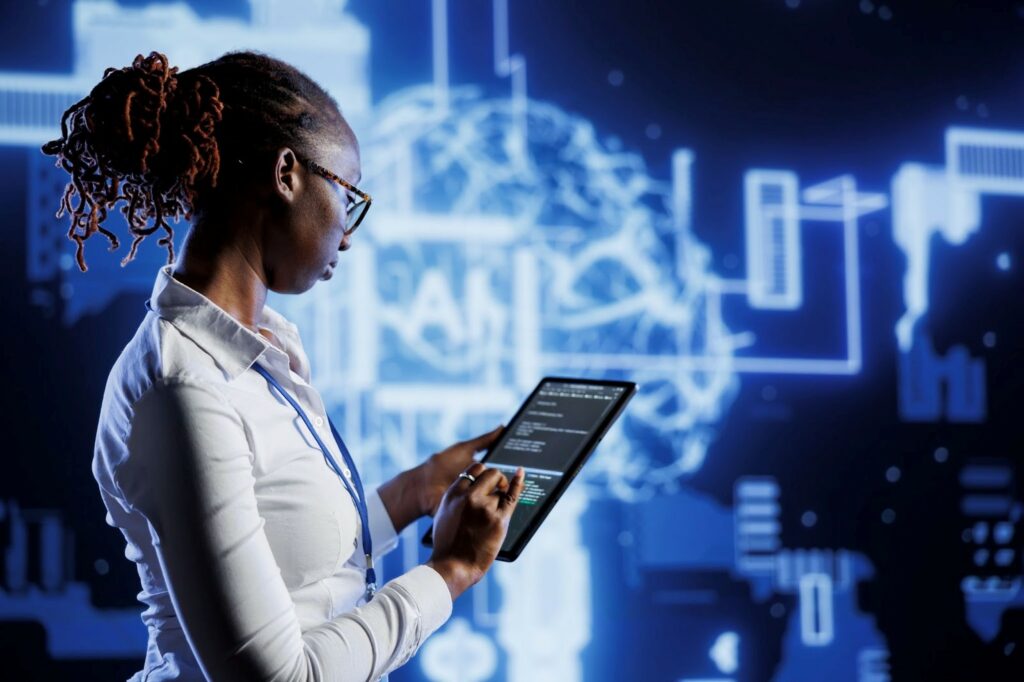
Generative AI is part of artificial intelligence (AI) where computers create entirely new content. Think of it as a computer version of famous artists like Picasso, Shakespeare, or Mozart, making entire creative pieces like texts, pictures, music, or even virtual worlds.
Picture having an AI friend who excels at spinning tales. Just give it a prompt like “Wandering through a mystical forest…” and it crafts an engaging story with characters, plot twists, and an exciting ending.
This is how generative AI can take a simple idea and blossom it into a full story. And guess what? It’s not just limited to storytelling it can even paint, compose music and can even do coding.
Moving further, let’s shed some light on why generative AI has become the hot topic of discussion and headlines.
What’s All the Buzz About Generative AI, and Why Is Everyone Talking About It?
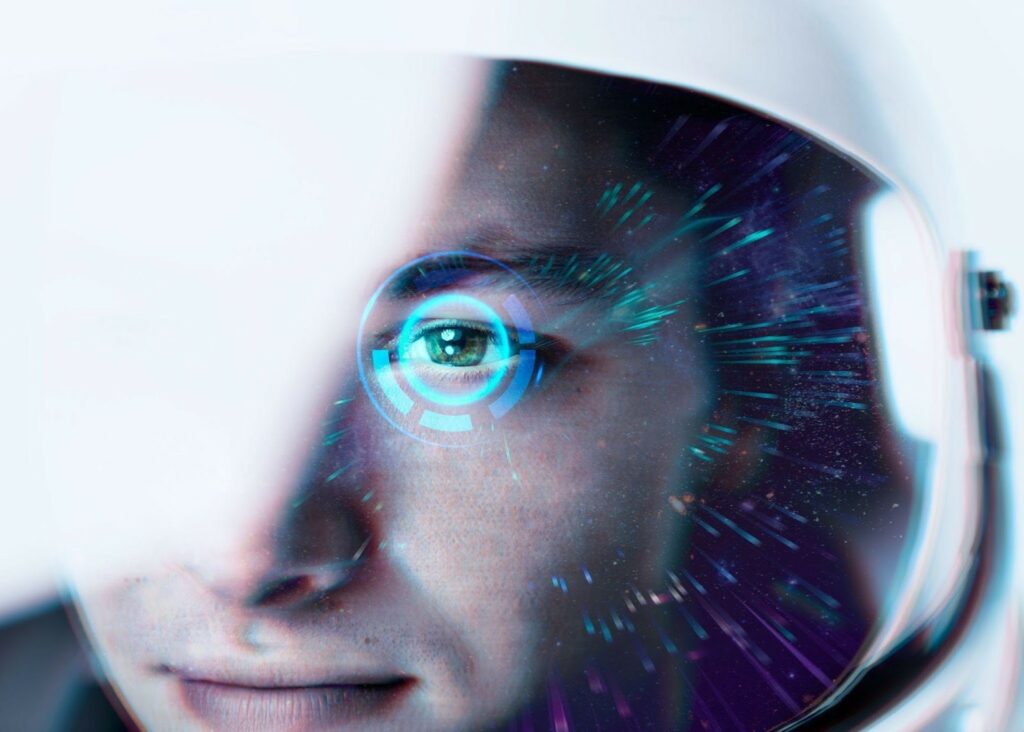
In 2023, generative AI took the spotlight, dominating headlines and earning its status as a ‘must-have’ technology for businesses.
But why this buzz? Why generative AI is on the lips of every business owner? The reason behind this is: Generative AI possesses the potential to entirely revolutionize the work process.
It holds the potential to streamline tasks that presently consume considerable employee resources, affecting industries ranging from banking to life sciences. Generative AI is different from temporary trends like cryptocurrency and the metaverse. It’s here to stay in the business world and ready to make its mark across different industries.
However, despite its potential, numerous enterprises find it difficult to adopt this new technology. This hesitation comes from understanding that making these technologies work takes more effort than just reading and understanding about them.
The main obstacle is to bridge the gap between the eagerness to adopt generative AI and the competency to effectively embed and enhance its utilization within the operational setups of businesses.
After recognizing the excitement surrounding generative AI, let’s shift our focus to understanding the scientific principles that facilitate its operation.
What Scientific Principles Drive Generative AI’s Ability to Generate New Data?
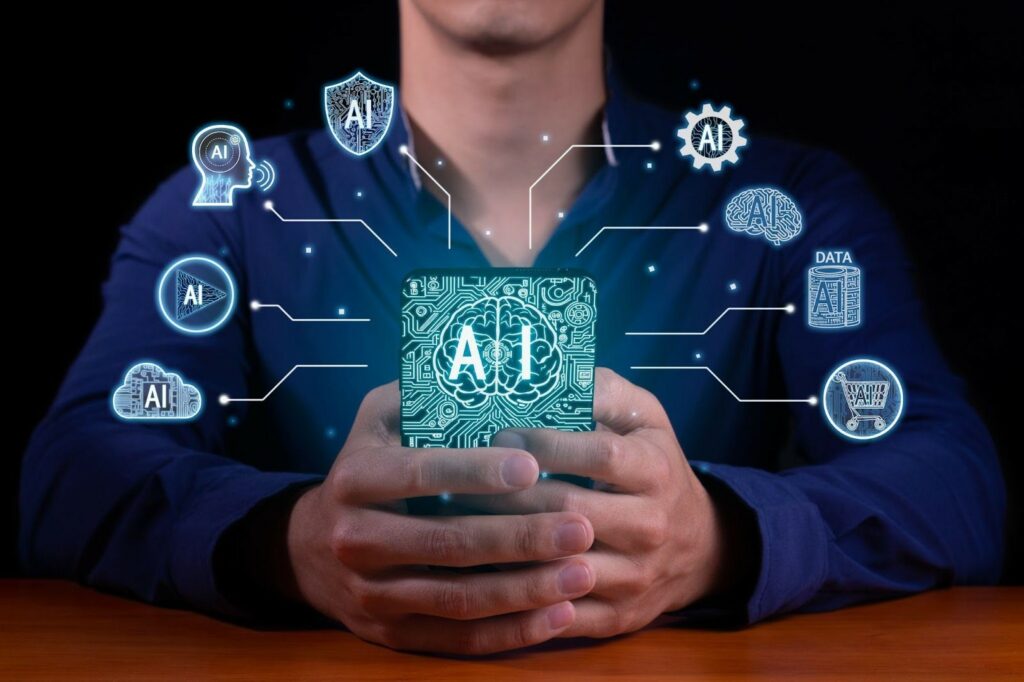
- Generative AI utilizes Generative Adversarial Networks (GANs) to create new data samples like images or text.
- GANs involve two neural networks: the generator and the discriminator.
- The generator network generates data samples from random noise.
- The discriminator network distinguishes between real and generated data.
- Through a competitive feedback loop, the generator improves its output to mimic real data better.
- Simultaneously, the discriminator enhances its ability to detect artificial content.
- Generative AI has diverse applications in art, music, medicine, and beyond.
- The training process fosters continuous improvement, resulting in the generation of increasingly realistic data samples.
- This iterative process leads to the production of high-quality data that closely resembles real data.
- Applications of this technique include image and speech generation, demonstrating its effectiveness in producing lifelike and diverse content.
Let’s delve further into the topic and shed some light on what is the difference between generative AI and AI.
Generative AI vs.Traditional AI
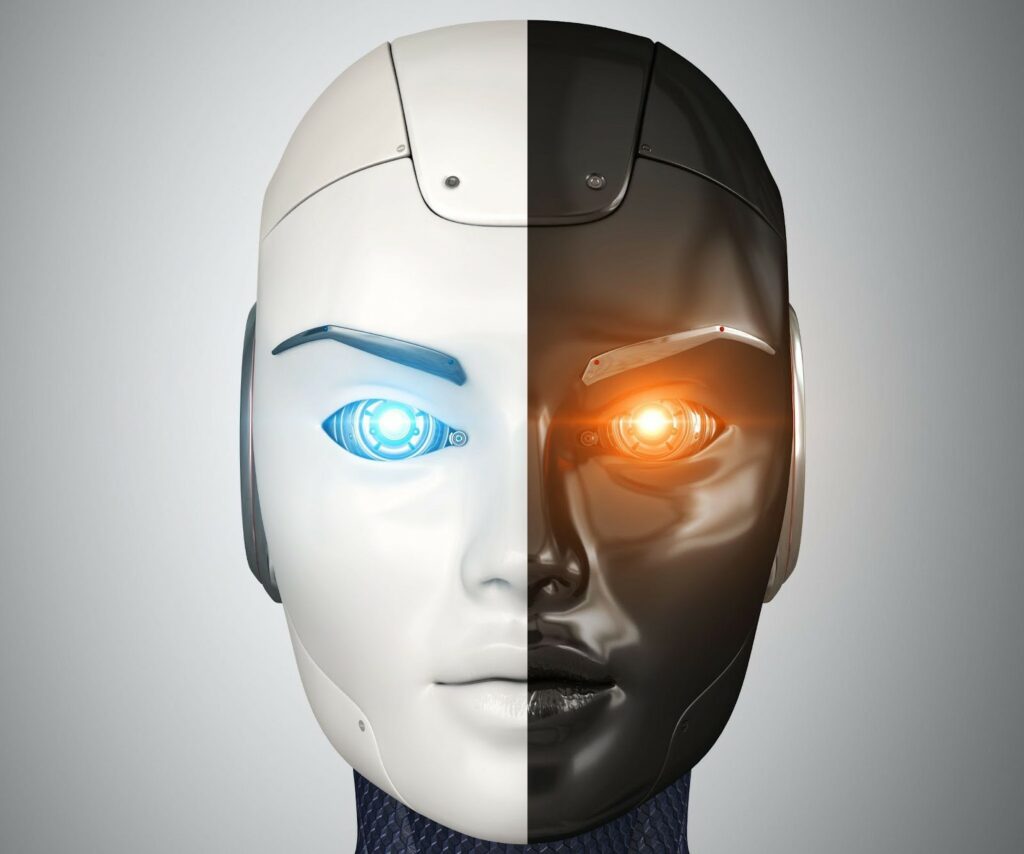
Let’s understand the difference between the topics with a tabular comparison.
Parameters | Generative AI | Traditional AI |
Objective | Creates original content or data from scratch. | Perform predetermined tasks according to established guidelines. |
Learning Approach | Autonomous learning | Learning guided by supervision or predefined regulations. |
Data requirements | Relies on extensive datasets for training. | Requires labeled datasets for training. |
Problem-Solving | Handles open-ended problems. | Deals with particular, well-specified problems. |
Creativity Abilities | Generates distinctive and individualized artwork and designs. | Lacks built-in creative abilities. |
Anomaly Detection | Pinpoints irregular patterns or events. | Follows predetermined criteria for anomaly detection. |
Examples | GPT-3, DALL-E | Expert systems, and rule-based systems. |
Having explored the contrasts between traditional and generative AI, let’s embark on unraveling the myths encircling generative AI.
Common Myths Surrounding Generative AI
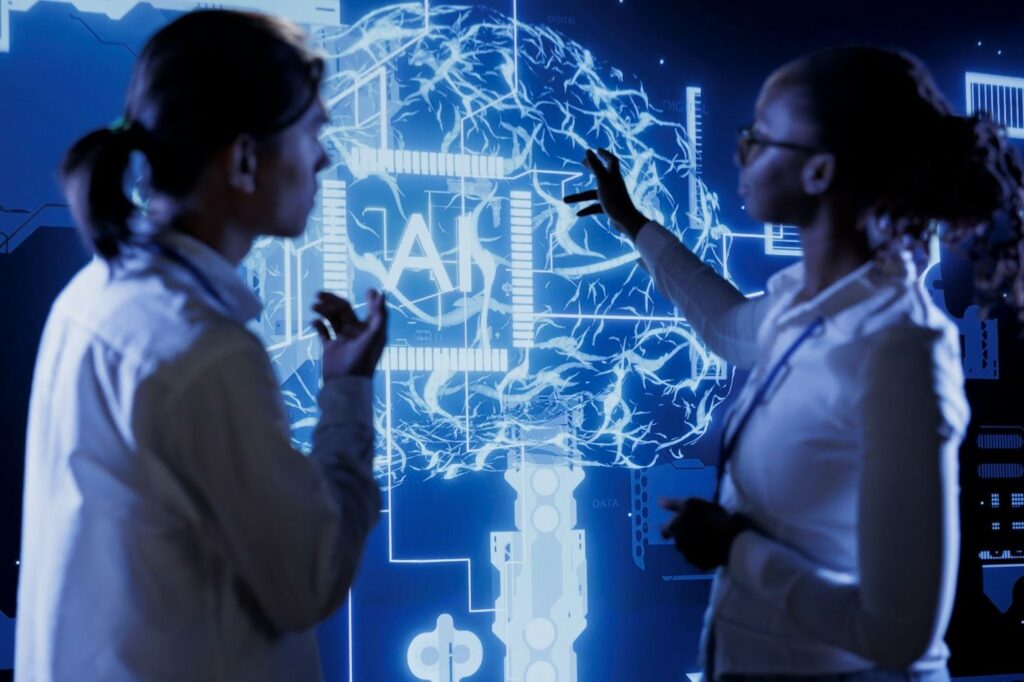
Here are some of the most common myths surrounding generative AI. Let’s have a look at them.
- Generative AI sparks fear among individuals for losing their jobs.
- People doubt generative AI’s caliber to produce high-quality content.
- Privacy and security hang in the balance as Generative AI gains momentum.
- Implementing Generative AI might be a hefty investment for small businesses.
- Human autonomy in decision-making could diminish due to Generative AI.
- Generative AI aims to substitute rather than enhance human skills.
- The replication of human behavior is effortless for Generative AI.
- Tech giants might dominate industries with Generative AI.
- Traditional art and creativity could face extinction with the rise of Generative AI
- Generative AI is poised to outshine human creativity.
These are the most common myths that people have regarding generative AI. Let’s now dig in to debunk some of these misconceptions while staying mindful of ethical concerns.
Unraveling the Truth of Generative AI Amidst the Myths Encircling It
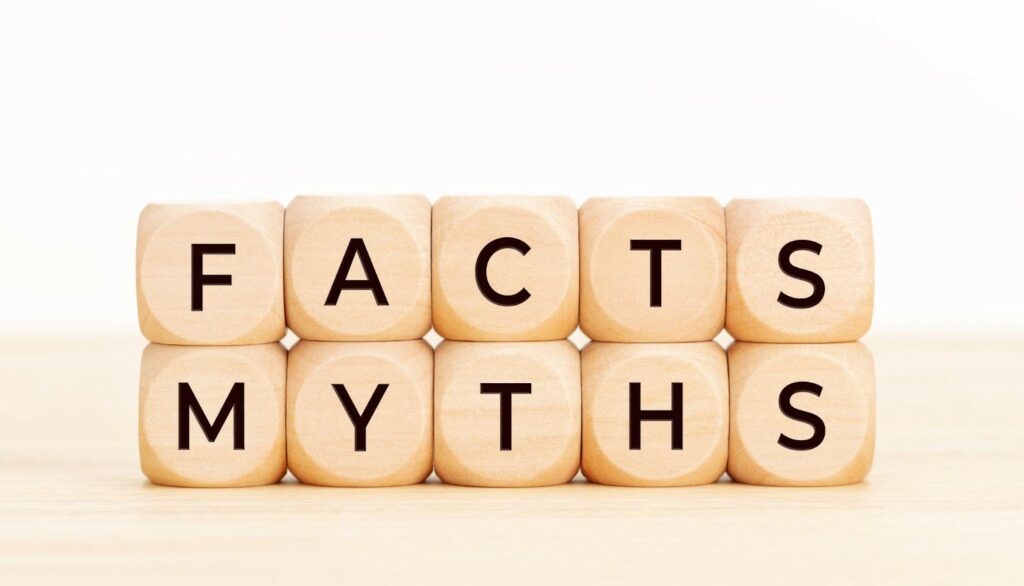
Just like every groundbreaking innovation, generative AI is surrounded by a whirlwind of misconceptions. Let’s unravel these myths and illuminate the transformative potential of this technology.
Myth 1: The Mistaken Idea of a One-Size-Fits-All Framework
The idea that one universal generative AI model can meet all needs is mistaken. Like the tech industry’s diverse array of companies, generative AI will likely consist of numerous models specialized for specific tasks.
Whether it’s summarization, list creation, or reasoning, different models excel in different areas. Additionally, industries and companies have unique editorial tones and knowledge expression requirements, thus requiring a variety of models.
Myth 2: Only Data Professionals Can Utilize Generative AI
Another common misconception is that generative AI is exclusively for data professionals or those with advanced technical skills. Although creating AI models involves complex algorithms and data manipulation, efforts are underway to simplify generative AI.
Today, the power of generative AI is accessible to individuals with various levels of technical expertise thanks to user-friendly platforms and tools. This accessibility promotes creativity in diverse fields like design, marketing, healthcare, and education.
As generative AI becomes more user-friendly, its potential applications will expand, providing a wider range of professionals access to its capabilities.
Myth 3: Generative AI’s Role in Education Raises Concerns About Enabling Plagiarism
Educational institutions, teachers and even parents are concerned about the impact generative AI might have on education. The reason for this worry is that people fear that generative AI will give rise to widespread plagiarism and compromised academic integrity.
Considering the immense capacity of AI to generate content, responsible usage of this technology requires ethical considerations.
Educational institutions are adopting this technology by instilling ethical practices among students along with incorporating advanced plagiarism detection tools.
The emphasis is on teaching people to use AI tools responsibly and highlighting the importance of being original and thinking critically.
When employed ethically, generative AI enriches the educational journey by fostering creativity, collaboration, and innovative thinking.
Myth 4: AI Doesn’t Rely on Human Oversight
AI systems possess considerable processing capabilities, allowing them to analyze vast data sets and make intricate decisions. Nevertheless, like any technology, especially new ones, AI systems have limitations and may encounter scenarios beyond their original design.
This is especially crucial in industries prioritizing safety regulations, quality standards, and user satisfaction.
Although AI algorithms can recommend products to customers, they don’t grasp cultural nuances or personal tastes as humans do. If the data used to train the algorithm is biased or incomplete, it might continue those biases or find it hard to adapt to new situations correctly.
In the near future, AI won’t work independently. Humans will need to regularly check, maintain, and train it to ensure it works accurately, safely, and ethically.
Myth 5: Generative AI Will Take Away All Human Jobs
This is a very common misconception rather it is a fear that has occupied human minds. Well, what we need to understand is that human talent is at a premium right now. Every organization or institution is more focused on cutting costs rather than jobs.
The issue isn’t about replacing but enhancing. Generative AI, in its current state, isn’t prepared to completely take over tasks or processes.
Yet, it proves to be a helpful partner in managing everyday or repetitive tasks, freeing up individuals to focus on essential and strategic parts of their duties. While it excels at handling tasks people might not enjoy, human oversight is essential.
Ultimately, it’s more like predicting the next word than making complex decisions.
Myth 6: Generative AI Ensures the Confidentiality of Shared Data
It’s crucial to be cautious when employing freely accessible models like ChatGPT, as they rely on data provided by users for ongoing improvement.
A well-known incident involving Samsung’s ChatGPT data leak highlights the dangers of sharing sensitive information, which can harm competitiveness.
To counter these risks, it’s advisable to ensure that any data shared with ChatGPT or similar models is already in the public domain, thus minimizing the potential impact of data exposure or unauthorized usage.
By being vigilant about data-sharing practices, businesses can prioritize data privacy and protect their confidential information effectively.
Myth 7: The Bigger the Model, The Better
There exists a widespread misconception surrounding the notion that the performance of a generative AI model is directly proportional to its size.
Bigger models have advantages like handling big data, but thinking that bigger is always better completely ignores the complexity of AI.
The efficacy of a generative AI model depends on numerous factors, encompassing the quality of the training data and the alignment of the model architecture with the specific task at hand.
In certain scenarios, smaller and more meticulously crafted models might surpass their larger counterparts in performance.
Therefore, it’s crucial to meticulously consider the balance between model size, computational resources, and practical effectiveness when developing and deploying generative AI systems.
Summing Up
As we enter the new year, let’s move past the misconceptions and instead concentrate on leveraging this innovative technology to craft tailored and enhanced customer experiences.
By strategically integrating AI with innate human capabilities and creativity, you can uncover pathways for growth and scalability that don’t necessitate significant initial investments in resources, time, or expenses.
If you feel any difficulty in understanding how you can make generative AI work for your business, feel free to contact us.