Are you struggling to grow and sustain your food and beverage business? Has your competition recently integrated AI tools into its business operations? Don’t worry, the generative and exponential power of AI in the food and beverage industry is the answer to your challenges!
Artificial Intelligence offers us tools that behave, think and solve problems like humans. In 2020, more than 20% of the F&B industry had integrated AI into their businesses. The figure is expected to reach 50% by 2023.
AI is already popular with winemakers, Fortune 500 companies, agriculturists and other actors in the F&B industry.
Let’s look at how the use of AI in the food and beverage businesses is making the industry more intelligent by the day…
Benefits of Using AI in the Food and Beverage Industry
As of 2023, the American food and beverage industry is worth $1.5 trillion and comprises 5% of the country’s GDP. However, natural calamities, fragile supply chains, diseases and other contingencies pose imminent challenges to its growth. AI offers a potent answer to such challenges—let’s examine how.
1. Delivering Food Security
Due to a rising global population, the demand for food will rise by 59-98% by 2050. But natural disasters – droughts, floods and forest fires – threaten crops, poultry and meat production worldwide.
AI is a trump card that can help solve this existential problem. Indeed, companies, farmers, governments and non-profits have all started leveraging AI tools to secure better yields.
Machine Learning, Spectroscopy and Computer Vision Systems have all been deployed to:
- Understand oscillating weather patterns,
- Monitor changing soil structures,
- Predict volatile price changes in supply chains and
- Diagnose evolving plant and animal diseases.
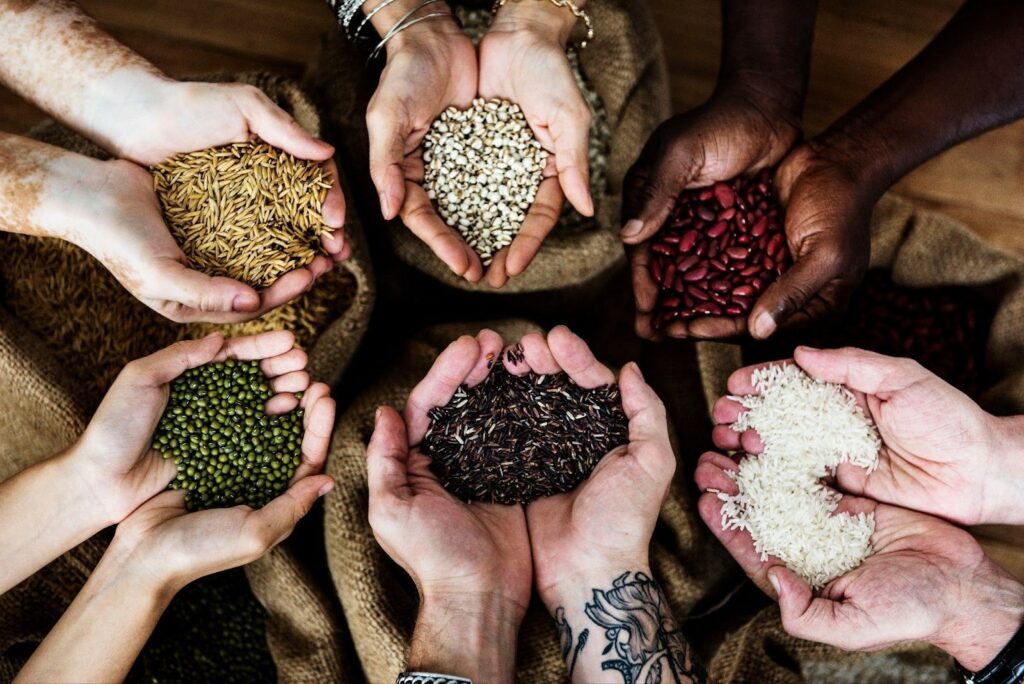
2. Ensuring Nutrition and Compliance
The fastest-growing F&B segment is plant-based and sustainable food. Companies want to translate scientific solutions into sustainable and organic products that provide nutritional value to their consumers.
Governments have also recognized the changing nutritional requirements of their populations. As a result, the FDA has enhanced compliance requirements for F&B manufacturers.
AI helps companies meet the FDA standards. Using expert systems, machine learning and vision systems, they can predict, record and convey the nutritional indicators present in their products.
AI tools like spectroscopy and neural networks can also help detect defects or deficiencies in seeds, cereals, alcoholic beverages and meat.
3. Streamlining Supply Chains
Global supply chains have transformed into cross-continent pipelines to meet increasing food demand. However, this trend is not without its challenges. The COVID-19 pandemic exposed the fragility of the global food supply system.
Fuzzy logic algorithms and ANNs can help make the global supply chain more robust. These tools can predict food shortages during pandemics, temperature rises, and weather disturbances. Such predictions can help prepare for wheat, wine, beer and more production.
AI thus provides alternatives that can save lives and fill stomachs during climate-driven starvation and shortages.
During non-emergency periods, AI can deliver efficiency and strengthen supply chains. An experiment revealed that a fuzzy logic algorithm reduced electricity consumption in a factory by 7%.
4. Enhancing Consumer Experience
Necessity has historically driven invention. Desire, on the other hand, has demanded innovation. Delivering quality consumer experience holds exponential growth potential for F&B operators.
This is especially true in consumer-facing businesses – AI in restaurants can be a game-changer. Imagine if AI can:
- Tailor new dishes to the preferences of repeat customers,
- Predict the recipes most likely to draw new customers,
- Convey to the customers the calorific values and nutritional figures of each dish,
- Automate targeted calls to ideal prospective customers,
- Receive and answer queries from customers so that you never miss a call!
Well, you don’t need to imagine! AI tools like fuzzy logic algorithms, machine learning and expert systems are available at your fingertips to transform the consumer experience you offer to another level while saving you money!
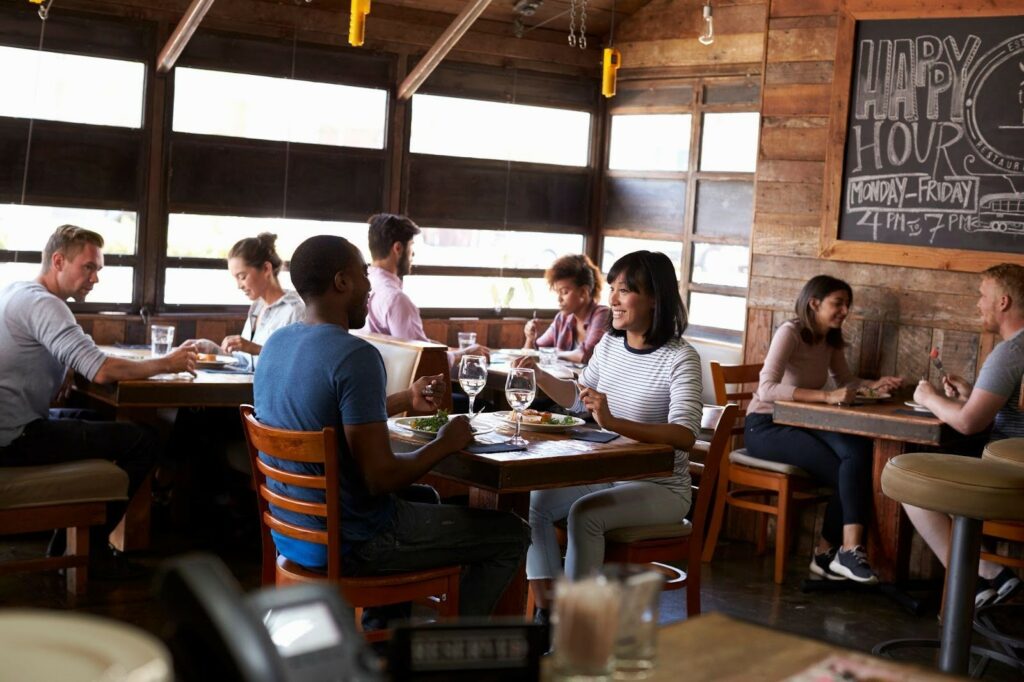
5. Driving Innovations
What new candy flavor will children like the most? Which ice cream flavor most entices the taste buds of your target market? Which wine would sell more – one with a hint of oak or one that spices up the people’s palettes?
Until now, companies have been at the mercy of clunky, expensive user surveys to answer such questions. Worse still, different people may provide arbitrary answers to questions that can decide the fate of your F&B company.
Now, you won’t have to rely solely on resource-draining exercises that deal in conjecture and rely on people’s goodwill toward your brand. AI is here to help you.
With e-nose and e-tongue systems, neural networks and inference systems, you can invent the next popular beer in no time. Or you can design the perfect candy appropriate for all children.
Companies with deep-learning factories and facilities are changing how new products and food articles are developed. AI tools can thus help you transform your taste-testing processes into mini-Manhattan Projects!
Top Trends – How are Companies Utilizing AI in F&B Businesses?
Restaurant chains, mom-and-pop businesses, global F&B manufacturers, and local startups have all trusted AI tools to guide them into the future. Here’s how these enterprises harness the growing power of AI.
1. Innovating Rapidly
Food delivery apps and companies earn nearly $18 billion annually, and the four largest players boast over 80 million users on average. The industry is growing and shows no signs of slowing down.
Events like the Super Bowl, movie releases, music festivals and other entertainment avenues provide prospects for synergistic growth. After all, who attends these events and doesn’t eat out or order in?
By leveraging these opportunities, machine learning, ANN, FL algorithms, and expert systems can help maximize your company’s reach. These AI tools decrease the cost of acquiring new customers by telling you where and when to market your product.
With its learning capabilities, AI can tell you:
- Anticipate increasing demand,
- Deploy optimal capacity during specific times or days,
- Predict the largest clustering of prospective customers,
- Analyze trends in population density to determine avenues of expansion,
AI tools can improve conventional methods of understanding consumption trends to achieve targeted growth.
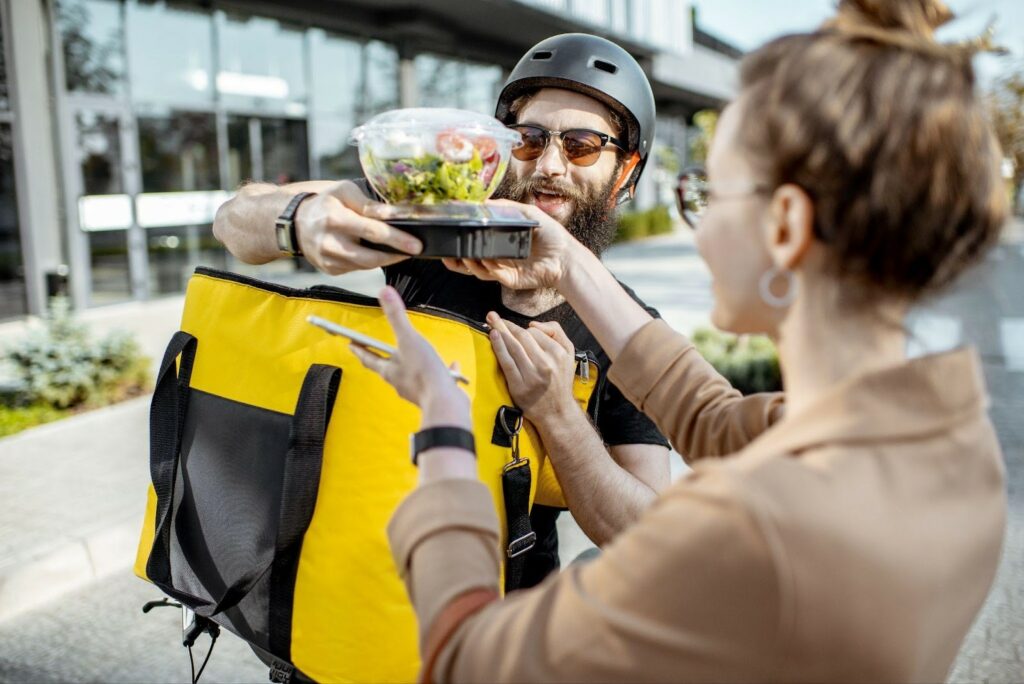
2. Adapting Technologically
Over 40% of food and beverage businesses have integrated AI tools to monitor external compliance mechanisms. They use them to:
- Determine the acceptability of their products,
- Sort food products by their segments and freshness,
- Predict expiration dates of their commodities,
- Virtually sample their prospective offerings to tailor them to specific tastes.
Over time, the profitability of production, acquisition, distribution and marketing processes in the F&B industry declines. Thus, companies must reduce costs and stay efficient to maintain lean business models.
AI tools like CVS and FL Algorithms help automate such processes. These tools analyze data points representing such operations. They show which processes are the clunkiest and how technology can best automate them.
3. Monitoring Defects and Deficiencies
Consumers are increasingly aware of what they eat and how it is produced. Ethical consumption is a hallmark of the contemporary customer. Companies must be vigilant about how their products are made and distributed throughout the marketplace. They aim to inspire trust and retain consumer faith.
76% of consumers say they value transparency in retail food shopping, an increase of 7% from 2018. The FDA has recently announced its plans to strengthen guidelines to ensure food quality and safety.
Companies have adapted to such changes by integrating AI tools to comply with these standards. They use spectroscopy, machine learning, vision systems and inference mechanics to:
- Detect the presence of harmful chemicals in beverages,
- Trace the contamination of meat products by harmful pathogens,
- Examine oxidation patterns in poultry and fish products and
- Diagnose potential defects in seed samples used to grow crops,
- Understand the effects of genetic modification on the resultant food products.
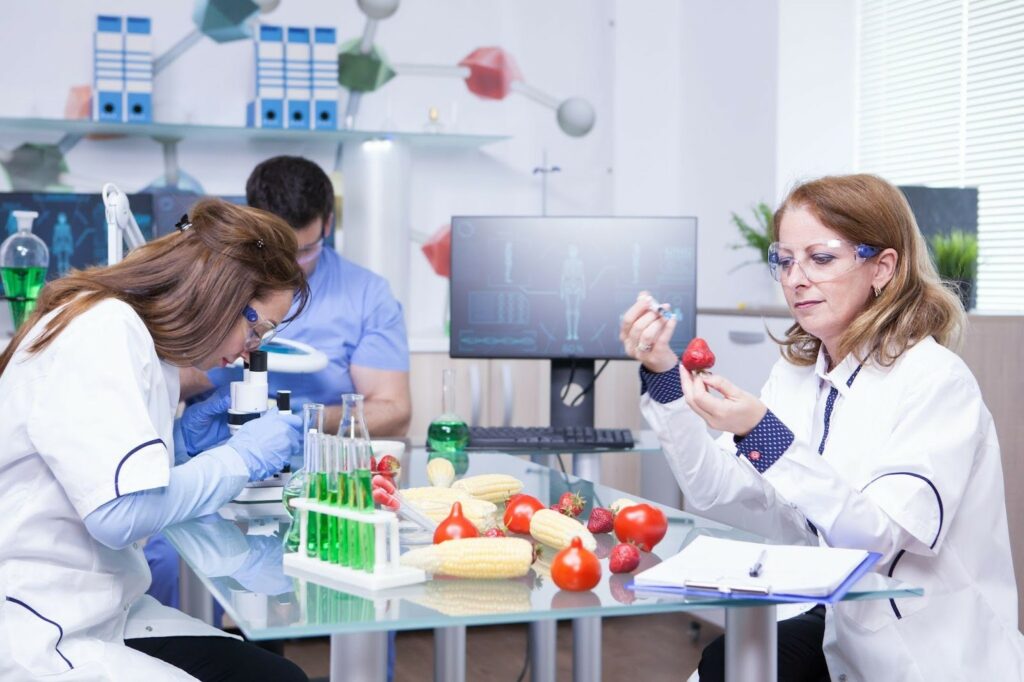
4. Data-Driven Marketing
Nestle has become the latest company to utilize generative AI and adapt their marketing strategies to its outputs. Generative AI is a machine learning model that creates new data based on existing patterns.
Companies such as Climax Foods are taking generative AI a step further. They trust it with:
- Creating frameworks for content marketing,
- Testing and creating new recipes,
- Personalizing consumer preferences,
- Generating plant-based alternatives to meat-based foods.
Aroma and taste testing and real-time analysis of food preferences are transforming the culinary experiences that F&B enterprises can provide. Thor Olf Philogene of Stravito says AI can identify potential customer’s pain points and turn them into hot leads for F&B companies.
5. Lean Business Processing
Every Super Bowl will feature new superfoods, but the underlying mechanical processes remain the same.
AI helps with both such processes. It reduces the steps required in an iterative process by automating it or showing areas where maximum costs can be cut. It has also helped companies modernize their internal communication processes and streamline supply chains.
On the other hand, generative AI reduces the time and effort required to develop new product ideas and strategies required to effectively market them.
If an AI model has understood your company’s history and practices, it will deliver recommendations for new products you can sell. All that’s left for you to do is eliminate the least feasible or effective recommendations.
All businesses deal in iterative processes:
- Procuring raw materials,
- Producing in fixed cycles,
- Marketing within budgets to specific segments and
- Distributing products through lean channels.
Here, the F&B industry is both an exception and a conformer. It is an exception because it must:
- Drive new culinary experiences,
- Offer seasonal alternatives and
- Refine existing options to stay competitive.
Avocados From Mexico, a not-for-profit company, created an AI tool, GuacAImole, that used image recognition, text and generative AI to create personalized recipe recommendations to add to the hype of the Super Bowl.
Thus, using AI will make your business operation lean. It will help you direct your precious human resources in pre-tested directions developed based on recent trends in consumer preferences.
Top 9 AI Tools Transforming the Food and Beverage Industry
AI in food and beverage businesses is not a monolith – it is a set of tools and services that automates, deploys and monitors:
- Food production cycles,
- Disease and pathogen detection regimes,
- Global and fragile supply chains,
- Distribution and procurement processes and
- Marketing and sales strategies.
To this end, AI scientists and engineers have developed many learning processes. Let’s examine some of these emerging technologies so you can determine which tool offers the largest competitive advantage.
1. Expert Systems
Expert Systems AI has three main actors involved in automating business processes.
- A Human Expert who creates a knowledge base.
- A knowledge engineer with computing expertise who converts the knowledge base into an inference engine (say, a mobile application).
- An inference system that connects the user to the knowledge base via an interface.
The users simply feed in the requisite information and get an answer based on the expert knowledge stored in the base.
Use Case – Banana Production
Bananas are a globally consumed commodity. The economies of several Latin American countries depend solely on banana plantations and farming (Banana Republics). Several types of producers compete in this market: large corporations, large landholders, peasant smallholders and plantation workers.
The failure of a banana crop due to a wide-ranging disease threatens consumers with pathogenic diseases. It also threatens the livelihoods and profits of all involved in banana cultivation. AI helps banana planters and corporations:
- Detect weather patterns,
- Predict pest and locust movements,
- Diagnose diseases through physical signs on banana plants.
Thus, AI helps reduce and mitigate the effects of natural and social processes that threaten its production and distribution.
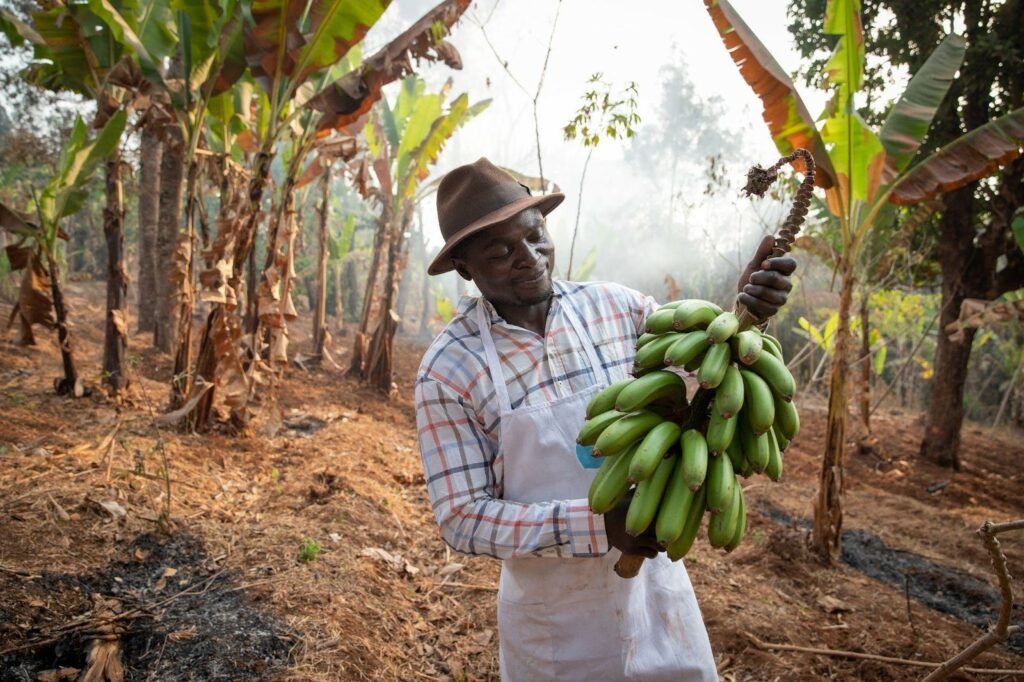
Example – Tumaini App
Banana planters and smallholders in Colombia, Indonesia, China, Congo (DR), Benin and other countries face periodic invasions of pests and diseases such as Xanthomonas, Fusarium wilt and Black Leaf Streak.
To solve this problem, the creators of the Tumaini App fed over 20,000 images of banana diseases and pests into an AI-based platform. Now, any farmer can simply log on to the app and scan images of plants, fruits or banana bunches to detect pest and disease infestation.
The app detects diseases and pest outbreaks with 90% accuracy and provides geospatial mapping and tracking of the phenomena.
2. Fuzzy Logic Algorithms
Zadeh introduced fuzzy logic algorithms in 1965. They are systems that convert absolute numbers into fuzzy categories. Based on these categories, they fuzzily transform the numbers using the IF/THEN principle.
For instance, this can be used to separate acid, basic and neutral chemical properties of various food products. The absolute pH numbers will be translated into fuzzy categories – 0-6, 7 and 8-14. Each product will fall into one of the three fuzzy categories. Once it falls into a category, we have to assign it an IF/THEN rule – if product A belongs to fuzzy category 0-6, then show output “Acidic” as the defuzzified value.
Use Case – Candies
Besides being used to reduce electricity consumption by adopting an FL model of temperature control rather than a conventional one, candy companies are also taking advantage of FL models to develop new flavors.
FL models can help break down nuances in candy flavors depending on the palettes of different segments and regions of customers. It can help distinguish candy flavors of the same type by regions that prefer a stronger flavor to ones that don’t.
For instance, a segment or region of consumers may prefer a stronger hint of cinnamon in their candies than others. With FL models, the flavors of the same candies can be adjusted regionally to deliver region-specific products.
Example – Mars Inc.
Mars Inc. has become the fourth largest privately owned corporation in the United States. It recently announced a strategic partnership with Microsoft and Accenture. They aim to create an AI Lab to streamline internal operations and predict consumer trends for effective product development. Mars also entered into a multi-year partnership with PIPA, an AI-based life science company, to accelerate the discovery of plant-based ingredients for human and animal food.
3. Artificial Neural Networks
Artificial neural networks offer problem-solving capabilities. They gain knowledge and learn through inter-neural connections based on synaptic weights. They can be used for pattern recognition and data classification.
For instance, they can classify combinations of cocoa beans that deliver a specific taste—bitter, sweet, salty, etc.
Use Case – Classification of Olive Oil
Scientists deployed ANN to test the variance between different types of olive oils. They based the variance on their chemical properties, locations and tastes. They made a database of 385 samples of Greek and Italian olive oils to do so.
An ANN analyzed the data to show that olives and olive oil samples differ in taste, composition and form depending on their geographical location and year of plantation.
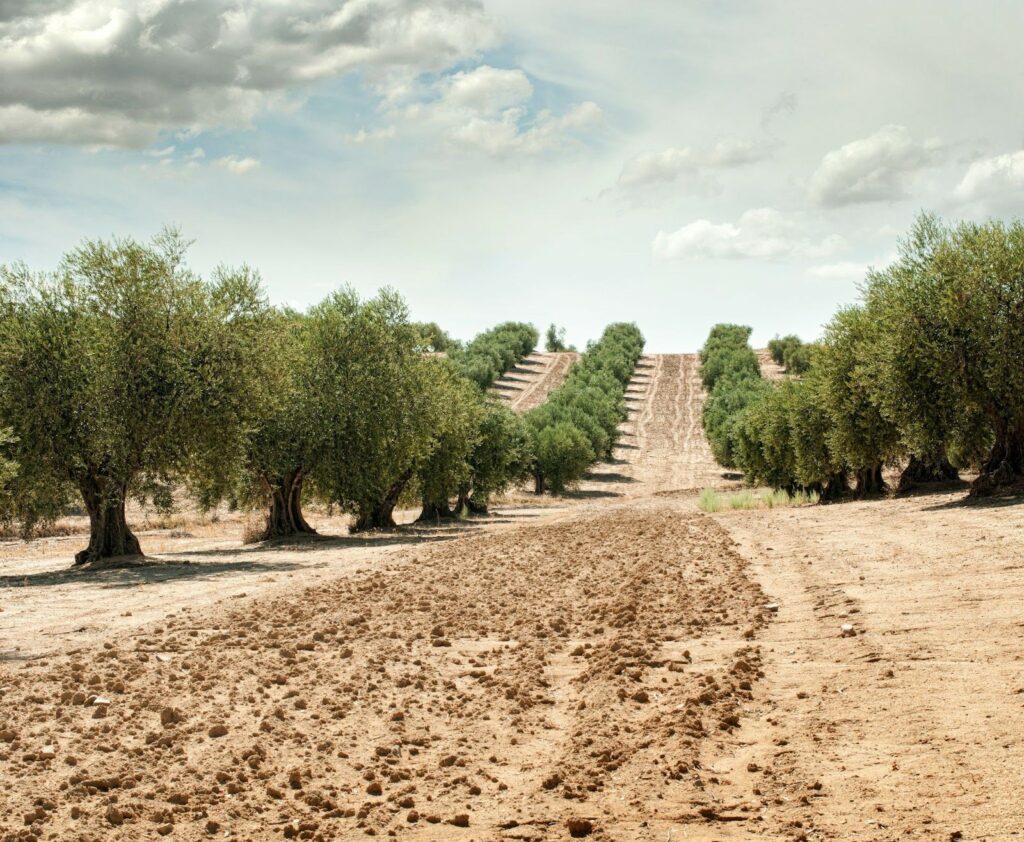
Example – Citoliva
Citoliva, a Not-for-Profit organization, has harnessed ANN to deliver a commercial solution to olive harvesting. It claims that the AI-based solution will deliver high yields and lower production costs.
The AI tool aims to “determine the optimal harvest moment without visiting the olive groves. It is designed to determine when the maximum amount of oil has accumulated within olive plants. It utilizes flowering, maturation, climate and satellite image data to achieve such results.
4. Machine Learning
Machine learning is the most popular AI tool used in automatic business operations. It learns automatically by experience. It can be classified into three types:
- Supervised learning – Through this, it understands specific patterns it is instructed to observe.
- Unsupervised learning – It observes naturally occurring patterns in a given data set or multiple data sets.
- Reinforced learning – It interacts between a program and an environment to determine patterns occurring within such interactions.
ML has several models—ANN is a type of ML tool. Other tools are regression analysis, decision trees, federated learning and more. A classic use of ML is to understand consumer behavior by looking at clustering patterns of users across geographical locations. It can then predict where a business’s next location can be set up to achieve maximum return on investment.
Use Case – Beer
MIT Technology Review recently reported how ML models can be fed data about beer tastes to reveal the most preferred beer palettes. A model was fed beer reviews from a popular beer review website and information about beer samples – hop, yeast and malt flavors.
After developing different ML models, the researchers adjusted the parameters of the beer based on the results. They then conducted blind test tastes of the resulting flavors – the beers “became better, and more generally appreciated.”
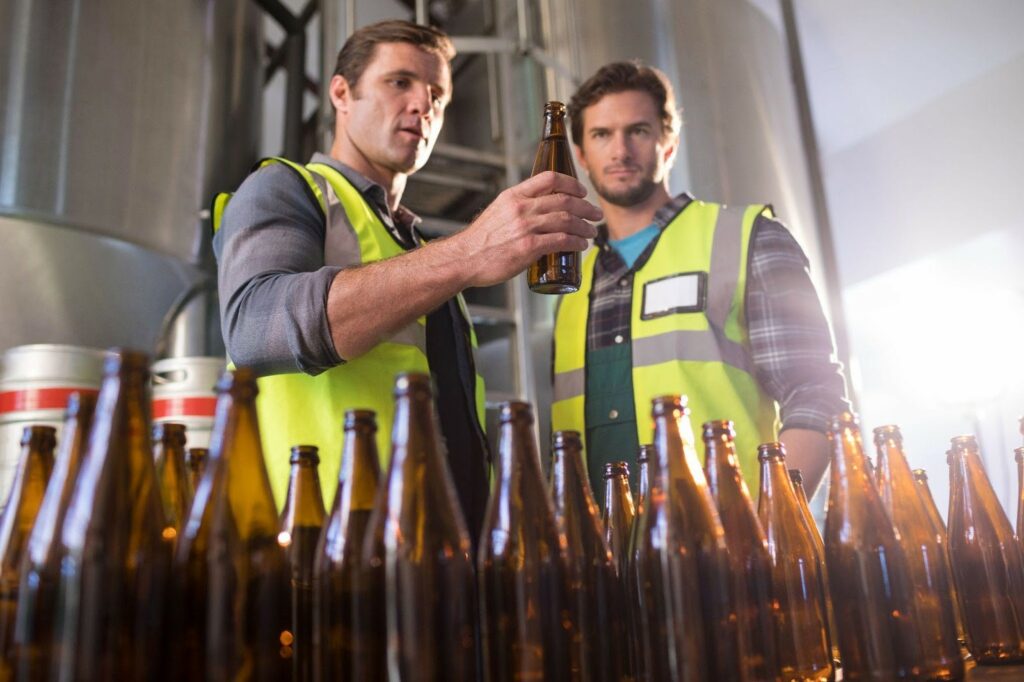
Example – Carlsberg
Carlsberg is the fourth-largest beer brewing company, with over 140 brands worldwide. In 2018, it led a new “beer fingerprinting project” in partnership with Microsoft and researchers from Aarhus University.
The research utilized machine learning, spectrometry and chromatography to reduce the time it takes to develop new beer brands by 30% while improving quality control. Nearly $4 million was invested in the project.
5. E-Nose
E-Nose utilizes machine learning, near-infrared spectroscopy and computer vision systems to sense different food and beverages. It functions based on an array of sensors and an intelligent algorithm to test samples and provide appropriate results.
Research suggests that e-noses have been used widely in quality control and assurance in the food and beverage industries. It has successfully tested wine, beer, dairy, meat, vegetables, fish, and more.
Use Case – Quality Control
Two scientists tested e-nose systems to detect food quality using artificial and convolutional neural networks and support vector machines. They used these systems to determine oxidation levels through gas sensors based on irreversible properties, such as the conduction of electricity through the resultant gasses.
Example – Nanyang Institute of Technology
Researchers at NIT, Singapore, have developed and tested an E-nose system that detects meat freshness with 98.5% accuracy. The system has an e-nose attached to meat packets and a barcode that displays the output.
The e-nose senses the gasses emitted by meat products when they decay, and the barcode changes color by the associated level of freshness. The system is further associated with a mobile application that reads the barcode and displays the predicted freshness of the meat products under surveillance.
6. E-Tongue
E-tongue utilizes several sensors and multivariate analysis to detect the presence of unknown and known liquids within a given solution. For instance, it can be deployed to milk to determine the impurity level.
It works based on a three-step process.
- First, the Sensors attached to the e-tongue acquire data,
- Second, the software or algorithm developed treats and sorts the data,
- Third, the E-Tongue compares and analyzes the data to determine fingerprints of desirable or undesirable chemicals.
Use Case – Quality Control and Experimentation
E-tongues have been used by the Japanese company Insent to conduct repeated quality-control tests and to describe various food and beverage products from a neutral perspective. The argument is that using an e-tongue rather than actual human taste tests has several advantages:
- Applicable expertise
Not everyone is an expert in everything. Wine sommeliers cannot test or taste coffee with equal efficacy. Knowledge bases are not transferable in human cases, whereas for AI, tastes are data points that can be translated into subjective sensations.
- Iterative Exercises
Quality control tests are iterative processes where precise standards must be met. Human taste tests cannot achieve similar results—something may taste acceptable but be masked by variegated chemicals to produce undesirable but detection-evading effects.
- Poisonous Samples
One of the major reasons for testing samples is to determine whether they are safe for human consumption. It is counterproductive for human beings to test such samples in the first place.
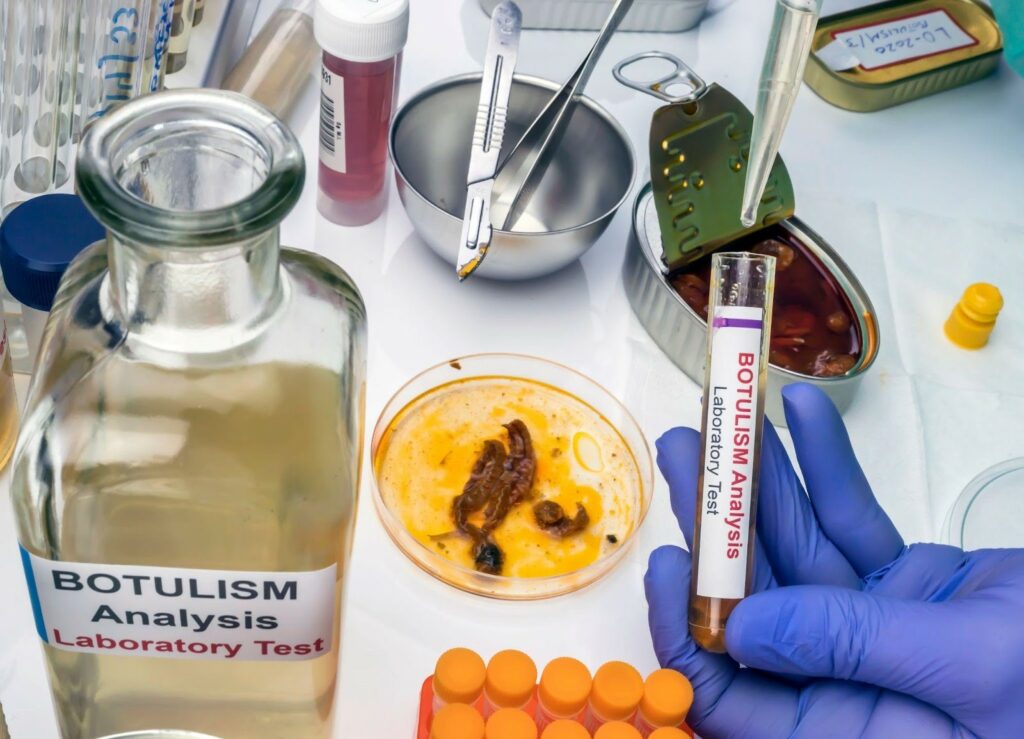
- Animal Food Tests
Several F&B businesses produce and manufacture pet foods. Again, it is counterproductive and inappropriate to put human subjects or sacrificial animals at the mercy of potentially harmful food products.
- Economic Reasons
Apart from the dangers of insurance and medical costs for food tests gone wrong, hiring several hundred or thousand taste-testers to scientifically and precisely measure different food objects for undesirable chemical components is not economical. An AI E-Tongue is much more effective and efficient for such purposes.
Example – IBM’s Hypertaste
In 2022, IBM revealed its Hypertaste E-Tongue, an award-winning chemical sensing solution capable of identifying complex chemical compounds in liquids and beverages. Its purpose and applications include sustainability, food science and health.
The array developed by IBM functions simply: you must dip it into a liquid. Its sensors react to the chemicals in the liquid to compare the sensations generated by the liquid and fingerprint them. A trained machine-learning algorithm then compares the sensations against a database of chemical compositions. After a chemical composition is identified, experts can delve into its specificity.
7. Computer Vision System
A computer vision system combines pattern recognition techniques with image recognition. It utilizes ANN and ML models to teach computers how to meaningfully understand patterns from images, videos and other visual media. It is commonly used to extract external features of food and beverage products, such as shape, size, color, consistency and defects. Three things comprise a CVS:
- A camera system for capturing the images of products,
- A lighting system to expose the features of the products,
- A software that processes and analyzes the captured images.
CVS techniques are used to achieve compliance with quality standards. They have also been deployed to:
- Describe the ripeness of bell peppers,
- Classify coffee beans,
- Predict the volume of eggs.
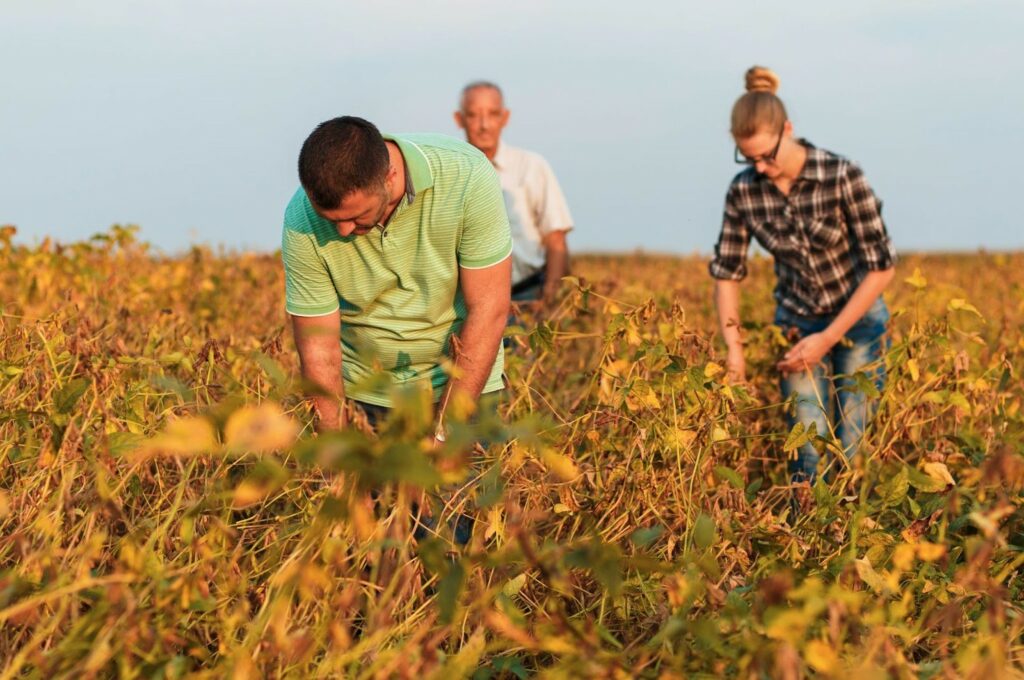
Use Case – Soybean Seed and Seedling Classification
Brazilian researchers used CVS to classify soybean seeds and seedlings according to their “appearance and physiological potential.” They developed a model combining images of soybean seeds and seedlings with free-access analysis software. After correlating the results with the seeds’ performance, they found that their model could accurately predict it with a 94% accuracy across all factors.
The researchers say their research was successful because soybean seeds’ appearance correlates with their performance. AI thus has applications in all F&B products in which performance and quality correlate with physiological appearances.
Example – Harvest CROO
Harvest CROO offers services for “crop management, harvesting and picking specialty crops.” According to its CTO, Bob Pitzer, the company began with the aim of automating Strawberry harvests. Strawberry cultivation faces huge challenges due to a lack of harvesting labor, as it is a labor-intensive crop.
However, they successfully automated berry harvesting using LiDAR and Differential Global Positioning Systems for robotic harvester navigation. They used AI-driven sensors that utilize machine vision to inspect which berries were ripe for harvesting. Pitzer adds that as the technology matures, its cost will reduce, making it an asset to farmers.
8. Near Infrared Spectroscopy (NIRS)
NIRS is a nondestructive and noninvasive tool that “continuously monitors regional oxygenation.” It can detect harmful toxins and pathogens by analyzing the chemical compositions of samples without destroying or disturbing them. NIRS is a cost-effective, simple and fast alternative to traditional methods that are expensive and time-consuming.
It functions in the following steps:
- It absorbs light reflected off samples,
- Reflected data is sent for processing,
- Processing reveals the presence of pathogens and chemicals.
NIRS has been used to:
- Determine the characteristics of controlled-processing cheese,
- Classify different types of food powders,
- Predict the quality of wheat flour.
Use Case – Cheese Quality Monitoring
Danish researchers used NIRS and Proton Nuclear Magnetic Resonance techniques to monitor the quality of semi-hard cheeses. They aimed to analyze the biochemical reactions and microbes that ripen cheeses such as Gouda, Cheddar and Emmental.
The research focused on analyzing 48 commercial semi-hard cheese samples. NIRS effectively showed the discriminative characteristics of cheese samples based on microbial cultures without physically invading the samples.
The findings show that NIRS tools can produce dairy and cheese while maintaining consistency, texture, chemical profile and taste.
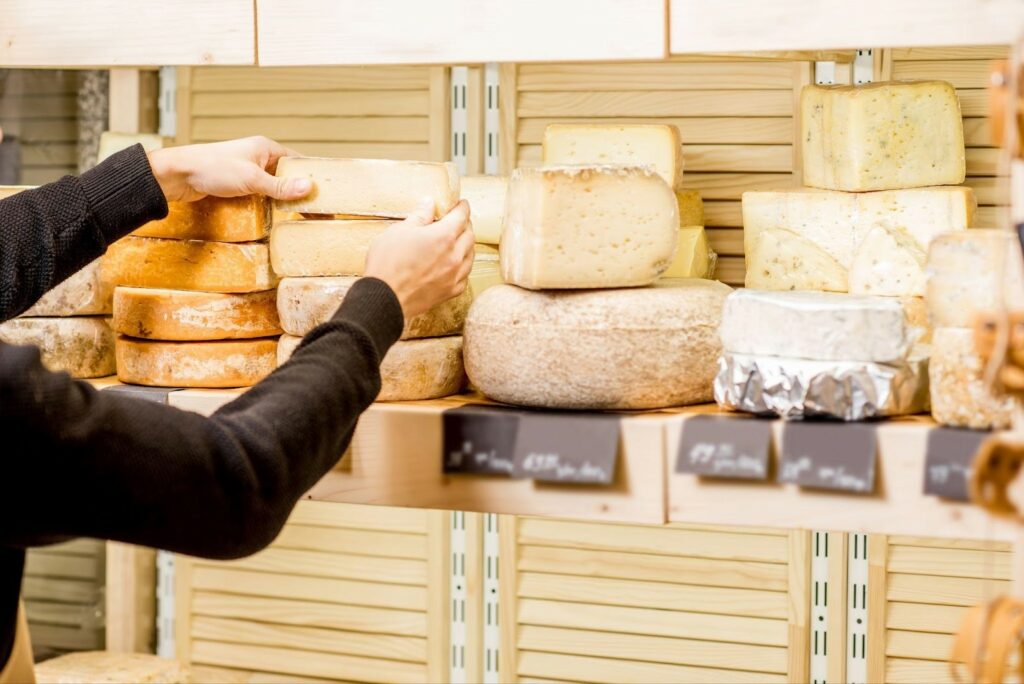
Example – Ametller Origen
Yahoo recently reported that Ametller Origen, a Catalonia-based business, has partnered with NeoSpectra by Si-Ware to develop NIRS solutions for on-site testing problems. In its first phase, they will develop models to test the quality of peaches, nectarines, strawberries and apricots.
Ametller aims to drive sustainable food production and distribution of high-quality foods through its 140+ European stores. An associated objective is to minimize food waste and maximize the commercial success of its produce. Through their collaboration, Ametllar and Si-Ware show how NIRS and AI can transform the landscape of culinary compliance and quality control.
9. Adaptive Neuro-Fuzzy Inference System
ANFIS is a hybrid AI system that combines Fuzzy Logic systems and Artificial Neural Networks. It combines human reasoning and computational power in one tool and is an alternative to conventional modeling techniques for interpreting complex relationships in large datasets.
It channels AI into the food and beverage industry in five major ways:
- Food drying,
- Prediction of food properties,
- Microbial growth and thermal process modeling,
- Applications in food quality control and
- Food rheology.
Research has revealed that it can be used to:
- Investigate the sensory attributes of ice cream,
- Optimize extraction conditions of antioxidants from Taro Flour
- Predict the taste of oranges and
- Determine moisture content in yams.
Use-Case – Food Production
European researchers combined ANFIS with Multilayer Perceptron tools to understand food production in Iran for over 50 years (1961-2017). They evaluated livestock and food production against livestock yield, live animals, and animals slaughtered.
The research revealed that an ANFIS model with generalized bell curve (Gbell) functions could accurately predict and model food production over time. They believe that policymakers can use the ANFIS AI tool to predict and strategize food production to achieve security and better yields in the future.
Example – Salmonella Detection
Several researchers from Iran and China used ANFIS and genetic algorithm-ANN models to study the activation of Salmonella Enteritidis in fresh-cut vegetables. They combined thermosensation, ultrasound and tea polyphenols to study fresh-cut gourds and estimate the presence of Salmonella bacteria in the samples.
Their research revealed a 98.6% accuracy in predicting Salmonella activation in the samples. It further revealed effective ways to treat Salmonella activation patterns using AI. Given the recent cases and a statistical increase in Salmonella cases in America, ANFIS techniques can be used to detect and prevent it efficiently.
Thus, AI tools in the F&B industry show great promise in cutting costs, increasing yields, making operations efficient and driving innovative solutions.
AI tools such as machine learning, fuzzy logic algorithms and computer vision systems can efficiently create, produce and monitor healthy food and beverages consumed by the American population. But can these tools withstand their challenges?
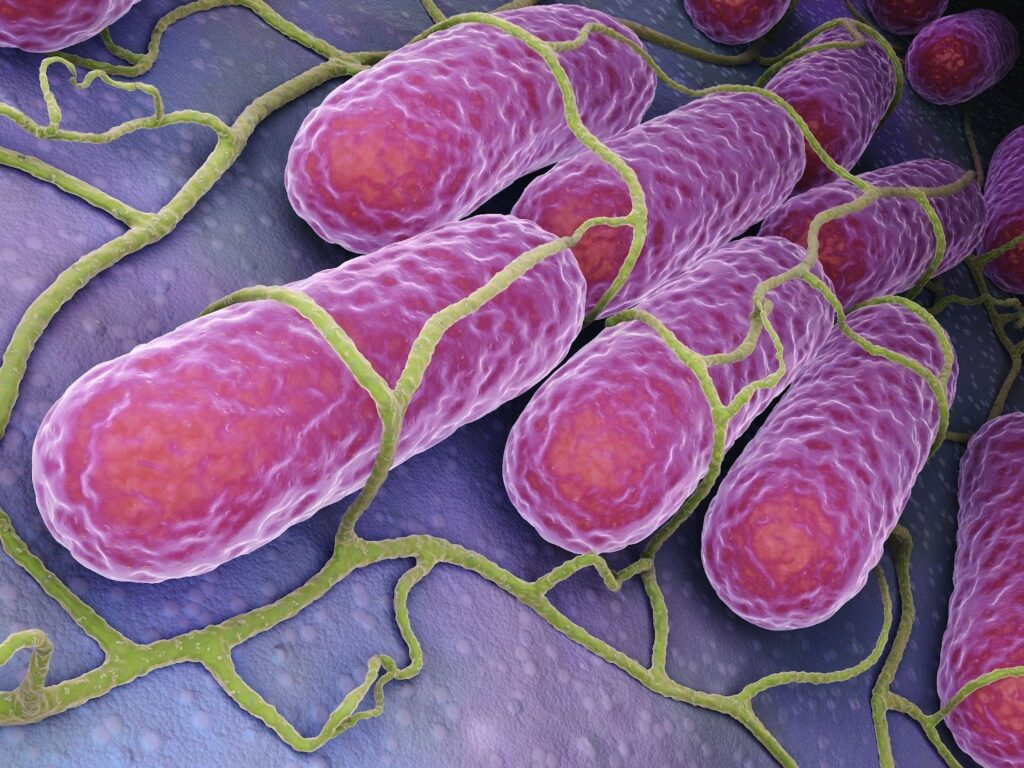
AI in the F&B Industry – What are the Challenges?
We have seen that AI can be key in unlocking potential gains in the food and beverage industry. It can strengthen supply chains, boost agricultural productivity and create innovative marketing and distribution models for up-and-coming food companies.
However, the use of AI is not without its own set of challenges. Let’s see what stands in the way of AI deployment in the food and beverage industry:
1. Implementation Cost
Companies developing AI solutions for the F&B industry must make the tools cost-effective. An e-tongue or an e-nose may be technologically feasible. However, their reach will be limited if they cannot make business processes cheaper.
Thus, AI solutions must become scalable, and innovation must not be prohibitively expensive. They must also help businesses deliver innovative solutions and cut operating costs. In the absence of this, AI is a bubble waiting to burst.
2. Cross-Industry Standardization
Fuzzy Logic algorithms and artificial neural networks cannot be standardized across industries. For instance, the best ice cream combines ideal viscosity, taste and weight. On the other hand, olive oil accumulation will depend on innate bio-physiological characteristics.
Thus, standardizing models to achieve scalability and ease of deployment can impede the application of AI to commercial F&B production. Governments and large corporations must share the costs of R&D needed to create AI tools that small businesses and manufacturers can use.
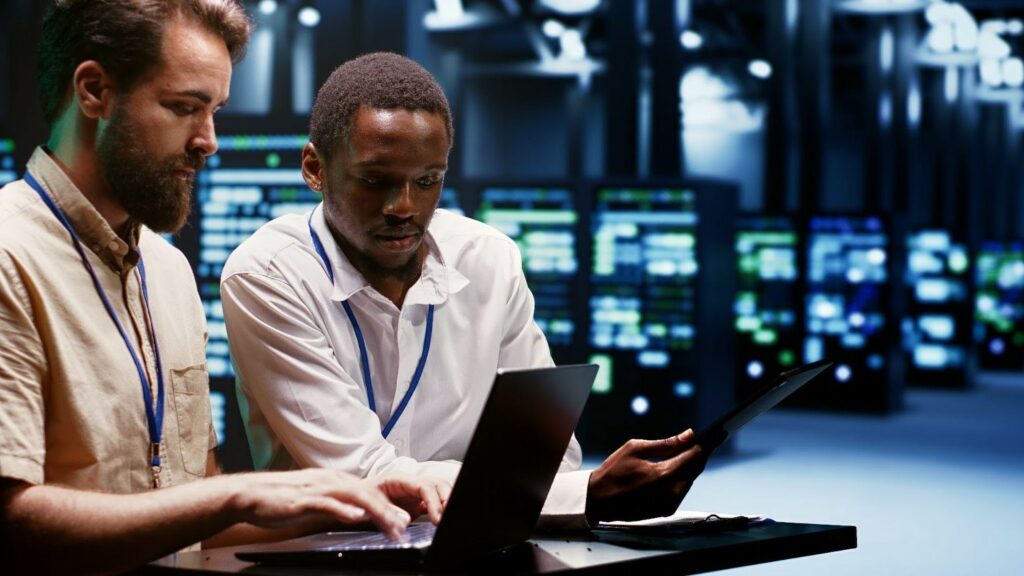
3. Data Collection and Security
AI relies on datasets and rules set by humans to interpret and apply to different samples. These rules and datasets must be safeguarded through best practices and government regulations.
Often, technology is developed and deployed, but rules and regulations take time to catch up. In the case of AI, regulations must accompany innovation because the datasets are stored on easily accessible cloud platforms and data banks. Without standardized security protocols, the very rules and data on whose basis AI tools operate are exposed to huge risks.
4. Translation to Business Practices
Tools like Artificial Neural Fuzzy Inference Systems are currently under development. They have yet to prove commercially viable. Similarly, E-nose and E-tongue systems have been developed with limited commercial applications.
Translating research into actual business practice is vital for the long-term needs of the F&B industry. The absence of commercial applications does not undermine such tools’ utility. Rather, it is a signal to the market. Whoever translates these technologies into business-friendly tools will retain a first-mover advantage and reap maximum profits.
Thus, AI tools’ challenges in the F&B industry are signs of huge potential commercial applications. Without risk, rewards are limited. AI is poised to change the way F&B businesses operate. The point of scalability and profitability is near, but companies must push for this change.
AI and F&B Industry – the Upward Trajectory
As of 2024, AI operations in the F&B industry are valued at $9.68 Billion. The figure is projected to reach nearly $49 Billion in the next five years. Chatbots, AI phone operators and generative AI have steadily grown in the past five years.
Further, 30% of all food and beverage products are discarded annually, amounting to losses worth $48 billion in revenue. Businesses are rapidly looking to cut these operational losses.
AI in restaurants can help drive up customers through generative marketing, whereas AI in F&B businesses can design efficient supply chains.
The use of AI tools in the F&B industry beacons. Integrating AI will soon become an attractive strategy to grow and survive in a competitive industry. At LeanSummits, we can help you seamlessly integrate smart AI technology tailored to your business needs and operations.
So why wait till AI goes mainstream? Get ahead of the curve and carve out a cutting-edge competitive advantage for your F&B startup!
Contact us and discover how the Age of AI can power your business…